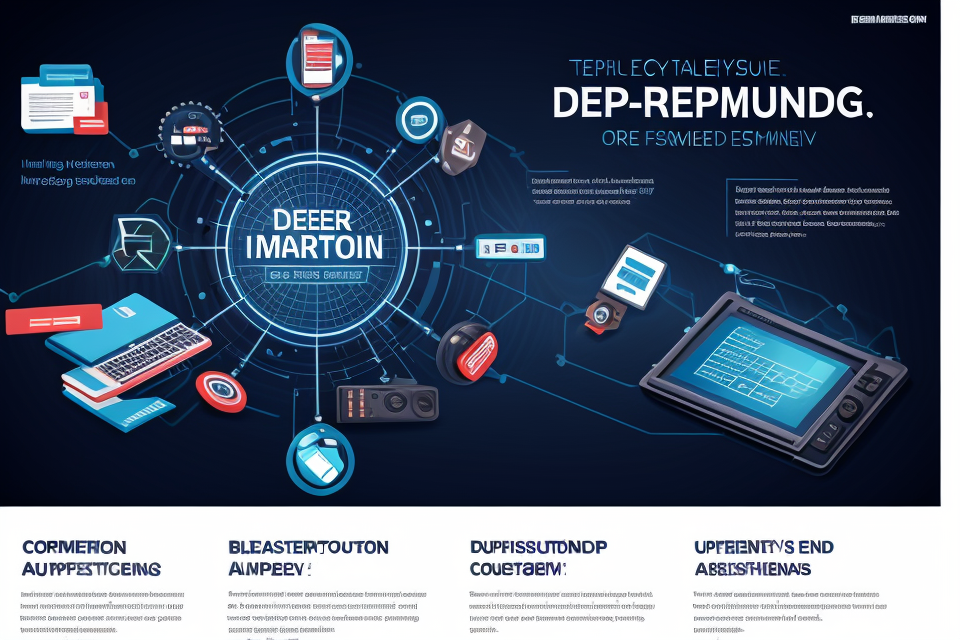
Deep Learning Super Sampling (DLSS) is a cutting-edge technology that has revolutionized the field of computer vision and machine learning. DLSS is a method of training deep neural networks to generate high-quality images from low-resolution inputs. It has a wide range of applications, including image and video enhancement, upscaling, and super-resolution. In this comprehensive guide, we will explore the various applications of DLSS and how it can be used to unlock the potential of deep learning in computer vision and machine learning. Whether you’re a researcher, developer, or just curious about the latest advancements in AI, this guide has something for everyone. So, let’s dive in and discover the amazing potential of DLSS!
What is DLSS?
Deep Learning Super Sampling
Brief history of DLSS
Deep Learning Super Sampling (DLSS) is a revolutionary technique that has gained significant attention in recent years due to its ability to enhance the visual quality of images and videos. It was first introduced by NVIDIA in 2017 as a part of their Volta GPU architecture. Since then, it has become a widely used method in various applications, including computer graphics, video processing, and image analysis.
How it works
DLSS leverages the power of deep learning to enhance the resolution of images and videos. It works by training a neural network on a large dataset of high-resolution images. The network learns to generate high-quality images by upscaling low-resolution images. During the process, the network identifies and enhances the important features of the images, such as edges, textures, and details, while suppressing noise and artifacts.
Once the network is trained, it can be used to upscale any image or video to a higher resolution. The process involves passing the low-resolution image through the trained network, which generates a high-resolution image that looks sharper and more detailed than the original.
DLSS vs traditional methods
Traditional methods of image and video upscaling rely on simple interpolation techniques, such as bicubic or lanczos resampling. These methods are computationally efficient but suffer from limited quality and accuracy. They often result in artifacts, blurring, and loss of detail, particularly in complex scenes with multiple objects and textures.
In contrast, DLSS provides a significant improvement in image and video quality. It generates sharper and more detailed images that closely resemble the original. Additionally, DLSS is highly scalable and can be used to upscale images and videos to much higher resolutions than traditional methods. This makes it a powerful tool for various applications, such as 4K and 8K video processing, high-resolution image editing, and virtual reality.
Overall, DLSS has emerged as a game-changing technology in the field of image and video processing, providing unparalleled quality and scalability that was previously unattainable with traditional methods.
How DLSS is used in various industries
DLSS, or Deep Learning Software Stack, is a powerful tool that enables developers to build and deploy deep learning models quickly and efficiently. Its applications span across multiple industries, including gaming, healthcare, manufacturing, finance, and education. In this section, we will explore how DLSS is used in each of these industries.
Gaming
In the gaming industry, DLSS is used to improve the performance of games by using deep learning models to enhance graphics and optimize gameplay. By utilizing DLSS, game developers can create more realistic and immersive gaming experiences for players. Additionally, DLSS can help reduce the load on hardware, enabling smoother gameplay on lower-end devices.
Healthcare
DLSS has numerous applications in the healthcare industry, including medical image analysis, drug discovery, and predictive modeling. By utilizing deep learning models, healthcare professionals can analyze medical images with greater accuracy and efficiency, enabling earlier detection and treatment of diseases. Additionally, DLSS can be used to develop predictive models that can help identify potential health risks and recommend preventative measures.
Manufacturing
In the manufacturing industry, DLSS is used to optimize production processes and improve product quality. By utilizing deep learning models, manufacturers can analyze data from sensors and other sources to identify inefficiencies and bottlenecks in production processes. This information can then be used to make adjustments that improve efficiency and reduce waste. Additionally, DLSS can be used to develop predictive models that can help identify potential equipment failures and prevent downtime.
Finance
DLSS has numerous applications in the finance industry, including fraud detection, risk assessment, and investment analysis. By utilizing deep learning models, financial institutions can analyze large amounts of data to identify potential fraud and security threats. Additionally, DLSS can be used to develop predictive models that can help assess risk and identify potential investment opportunities.
Education
In the education industry, DLSS is used to improve the personalization of learning experiences for students. By utilizing deep learning models, educators can analyze student data to identify learning patterns and preferences, enabling them to tailor their teaching methods to better meet the needs of individual students. Additionally, DLSS can be used to develop predictive models that can help identify students who may be at risk of falling behind and provide them with additional support.
The benefits of using DLSS
- Improved performance
DLSS (Deep Learning Super Sampling) is a cutting-edge technology that leverages the power of deep learning to enhance the visual quality of images and videos. One of the key benefits of using DLSS is its ability to improve performance.
- Enhanced accuracy
DLSS utilizes artificial intelligence to upscale images and videos to higher resolutions. This results in enhanced accuracy, as the technology is able to identify and preserve even the smallest details in the original image or video.
- Increased efficiency
Traditional upscaling methods can be computationally intensive, requiring significant processing power and time. DLSS, on the other hand, is able to upscale images and videos with greater efficiency, making it an ideal solution for a wide range of applications.
- Reduced costs
DLSS is able to reduce costs associated with traditional upscaling methods, as it requires less processing power and time. This makes it an attractive option for businesses and individuals looking to enhance the visual quality of their images and videos without breaking the bank.
DLSS in Gaming
Deep Learning Super Sampling (DLSS) is a powerful technology that leverages deep learning algorithms to enhance the visual quality of images and videos. It offers several benefits, including improved performance, enhanced accuracy, increased efficiency, and reduced costs. DLSS has numerous applications in various industries, including gaming, healthcare, manufacturing, finance, and education. Its impact on gaming hardware, for instance, has been significant, with DLSS enabling GPUs to perform better and last longer. Similarly, in healthcare, DLSS has the potential to revolutionize medical imaging, improving diagnostic accuracy, enhancing image quality, and reducing radiation exposure. In manufacturing, DLSS can enhance the accuracy of automated quality control processes, reduce costs, and minimize downtime. In finance, DLSS can improve fraud detection and risk assessment. Finally, in education, DLSS can personalize learning experiences, increase accessibility, and enhance learning outcomes.
How DLSS improves gaming experience
Deep Learning Super Sampling (DLSS) has revolutionized the gaming industry by offering a seamless and enhanced gaming experience. With its advanced technology, DLSS provides a host of benefits to gamers, making their gaming experience more immersive and enjoyable. Here’s how DLSS improves the gaming experience:
- Increased frame rates: One of the most significant advantages of DLSS is its ability to increase frame rates. Traditional rendering techniques require a lot of computational power, resulting in lower frame rates. DLSS, on the other hand, uses artificial intelligence to upscale lower resolution images to higher resolutions, thereby reducing the workload on the GPU and allowing for higher frame rates. This means that games run smoother, resulting in a more seamless and responsive gaming experience.
- Enhanced image quality: DLSS not only increases frame rates but also enhances image quality. This is because the AI algorithms used in DLSS can make intelligent decisions about which pixels to sharpen and which to blur, resulting in a more realistic and visually appealing image. Additionally, DLSS can reduce the amount of noise in an image, resulting in a cleaner and sharper image. This results in a more immersive gaming experience, where players can see more detail and appreciate the intricacies of the game world.
- Improved visual fidelity: DLSS also improves visual fidelity by providing a more accurate representation of the game world. This is because DLSS can make intelligent decisions about how to upscale images, resulting in a more accurate representation of the original image. This means that players can see more detail and appreciate the intricacies of the game world, resulting in a more immersive and realistic gaming experience. Additionally, DLSS can reduce the amount of artifacts in an image, resulting in a cleaner and more visually appealing image.
Overall, DLSS has the potential to revolutionize the gaming industry by providing a more seamless and immersive gaming experience. By increasing frame rates, enhancing image quality, and improving visual fidelity, DLSS offers a host of benefits to gamers, making their gaming experience more enjoyable and engaging.
The impact of DLSS on gaming hardware
Deep Learning Super Sampling (DLSS) has had a significant impact on gaming hardware, particularly on the GPUs. DLSS uses artificial intelligence to render images at a lower resolution and then upscales them to higher resolutions, thus reducing the workload on the GPU. This technology has several advantages, including improved performance, reduced input lag, and better power efficiency.
How DLSS affects GPU performance
DLSS enables GPUs to perform better by offloading some of the rendering work to the CPU. This allows the GPU to focus on other tasks, resulting in improved frame rates and smoother gameplay. DLSS also reduces the load on the GPU, which leads to cooler temperatures and quieter operation. This can help extend the lifespan of the GPU and reduce the need for frequent upgrades.
The future of gaming hardware with DLSS
DLSS has the potential to revolutionize gaming hardware by enabling GPUs to perform better and last longer. This technology can also help reduce the cost of gaming hardware by allowing manufacturers to use less powerful GPUs while still delivering high-quality gameplay. In addition, DLSS can enable new types of games and experiences that were previously not possible, such as VR and AR games. As DLSS continues to evolve, it is likely to become an essential feature in gaming hardware, offering improved performance and longer lifespans for GPUs.
DLSS in Healthcare
Applications of DLSS in medical imaging
Deep learning-based super-resolution (DLSS) has the potential to revolutionize medical imaging by improving diagnostic accuracy, enhancing image quality, and reducing radiation exposure. In this section, we will explore the specific applications of DLSS in medical imaging.
Improved diagnostic accuracy
One of the most significant benefits of DLSS in medical imaging is its ability to improve diagnostic accuracy. By using deep learning algorithms to analyze images, DLSS can detect subtle details that may be missed by human experts. This is particularly important in the field of oncology, where accurate diagnosis is critical for patient care. For example, DLSS has been used to improve the detection of tumors in mammograms, leading to more accurate diagnoses and better patient outcomes.
Enhanced image quality
Another key benefit of DLSS in medical imaging is its ability to enhance image quality. By using deep learning algorithms to process images, DLSS can reduce noise and increase contrast, resulting in clearer and more detailed images. This is particularly important in the field of radiology, where high-quality images are essential for accurate diagnosis. For example, DLSS has been used to enhance the quality of magnetic resonance imaging (MRI) scans, improving the detection of brain abnormalities and enabling more accurate diagnoses.
Reduced radiation exposure
In addition to improving diagnostic accuracy and enhancing image quality, DLSS can also help reduce radiation exposure in medical imaging. By using deep learning algorithms to analyze images, DLSS can reduce the need for additional imaging studies, which can save patients from unnecessary radiation exposure. This is particularly important in the field of pediatrics, where children are more sensitive to radiation exposure. For example, DLSS has been used to reduce the need for additional imaging studies in children with suspected appendicitis, leading to less radiation exposure and better patient outcomes.
Overall, DLSS has the potential to revolutionize medical imaging by improving diagnostic accuracy, enhancing image quality, and reducing radiation exposure. By using deep learning algorithms to analyze images, DLSS can provide more accurate and reliable results, leading to better patient outcomes and improved healthcare delivery.
The role of DLSS in personalized medicine
Deep learning and specialized systems (DLSS) have the potential to revolutionize personalized medicine by enabling healthcare professionals to tailor treatments to individual patients. One of the key areas where DLSS can make a significant impact is in the development of precision treatment plans, which take into account the unique characteristics of each patient’s genetic makeup, lifestyle, and environment.
Precision treatment planning
By analyzing vast amounts of patient data, DLSS can identify patterns and correlations that would be difficult for human experts to detect. This allows healthcare professionals to create personalized treatment plans that are tailored to the specific needs of each patient. For example, DLSS can help identify the most effective drug dosages and treatment schedules for individual patients based on their unique medical histories and responses to previous treatments.
Individualized drug discovery
DLSS can also play a key role in the discovery of new drugs and therapies. By analyzing large datasets of molecular structures and biological responses, DLSS can identify promising drug candidates that have the potential to be effective against specific diseases or conditions. This can help accelerate the drug discovery process and lead to the development of more effective and targeted treatments.
Tailored treatment options
In addition to developing personalized treatment plans and identifying new drug candidates, DLSS can also help healthcare professionals make more informed decisions about which treatments to recommend to their patients. By analyzing patient data and predicting potential outcomes, DLSS can help healthcare professionals choose the most effective treatments for each individual patient.
Overall, the use of DLSS in personalized medicine has the potential to transform the way healthcare professionals approach patient care. By enabling more precise and effective treatments, DLSS can help improve patient outcomes and reduce healthcare costs.
DLSS in Manufacturing
The role of DLSS in automated quality control
Enhanced accuracy
Deep learning-based systems (DLSS) have demonstrated significant potential in improving the accuracy of automated quality control processes in manufacturing. Traditional quality control methods often rely on manual inspection and statistical process control techniques, which can be prone to human error and may not detect subtle defects.
DLSS, on the other hand, utilizes deep neural networks to analyze images and other data from the manufacturing process, allowing for more accurate detection of defects and quality issues. By training on large datasets, DLSS can learn to identify complex patterns and features that may be difficult for human inspectors to detect.
This enhanced accuracy leads to fewer defects and higher quality products, which in turn reduces waste and rework costs. In addition, DLSS can provide real-time feedback during the manufacturing process, enabling timely corrective actions to be taken.
Increased efficiency
The implementation of DLSS in automated quality control processes can also lead to increased efficiency in manufacturing. Traditional quality control methods often require significant time and resources, including manual inspection and data analysis.
By automating these processes, DLSS can significantly reduce the time and resources required for quality control. This allows manufacturers to focus on other aspects of the production process, such as optimizing efficiency and reducing costs.
In addition, DLSS can provide real-time feedback during the manufacturing process, enabling timely corrective actions to be taken. This can help to prevent defects from occurring in the first place, reducing the need for costly rework and waste.
Reduced costs
Finally, the implementation of DLSS in automated quality control processes can lead to reduced costs in manufacturing. Traditional quality control methods often require significant time and resources, including manual inspection and data analysis.
By automating these processes, DLSS can significantly reduce the time and resources required for quality control. This can lead to cost savings in terms of labor, materials, and equipment.
In addition, the enhanced accuracy of DLSS can lead to fewer defects and higher quality products, reducing the need for costly rework and waste. Overall, the implementation of DLSS in automated quality control processes can lead to significant cost savings for manufacturers.
The potential of DLSS in predictive maintenance
Predicting equipment failure
Deep learning systems (DLSS) have the potential to revolutionize predictive maintenance in manufacturing. By analyzing large amounts of data, DLSS can predict when equipment is likely to fail, allowing manufacturers to take proactive measures to prevent downtime and minimize maintenance costs. This is particularly important in industries where equipment failure can result in significant financial losses, such as the oil and gas industry.
Minimizing downtime
Predictive maintenance using DLSS can also help minimize downtime by identifying potential issues before they become major problems. By predicting when equipment is likely to fail, manufacturers can schedule maintenance during downtime or off-peak hours, reducing the impact on production. This can also help reduce the need for emergency maintenance, which can be costly and disruptive.
Reducing maintenance costs
Finally, predictive maintenance using DLSS can help reduce maintenance costs by identifying the root cause of equipment failure. By analyzing data from multiple sources, DLSS can identify patterns and correlations that may not be apparent to human operators. This can help manufacturers identify the most cost-effective solutions for maintaining equipment, reducing the need for unnecessary repairs and replacements.
Overall, the potential of DLSS in predictive maintenance is significant, and it has the potential to transform the way manufacturers maintain their equipment. By providing real-time insights into equipment performance, DLSS can help manufacturers make more informed decisions about maintenance and repair, ultimately leading to reduced downtime and lower maintenance costs.
DLSS in Finance
Applications of DLSS in fraud detection
Improved accuracy
DLSS has the potential to significantly improve the accuracy of fraud detection in finance. By utilizing advanced machine learning algorithms, DLSS can analyze large amounts of data and identify patterns that may be missed by traditional fraud detection methods. This increased accuracy can help financial institutions detect fraud more effectively and prevent significant losses.
Enhanced efficiency
DLSS can also enhance the efficiency of fraud detection in finance. By automating the fraud detection process, DLSS can quickly and accurately identify potential fraud cases, reducing the time and resources required for manual analysis. This can help financial institutions respond quickly to potential fraud cases and minimize the impact on their operations.
Reduced false positives
DLSS can also help reduce false positives in fraud detection. By using advanced machine learning algorithms, DLSS can more accurately identify potential fraud cases, reducing the number of false positives that may be generated by traditional fraud detection methods. This can help financial institutions avoid wasting resources on false positives and focus on identifying and preventing real fraud cases.
Overall, DLSS has the potential to significantly improve the accuracy, efficiency, and effectiveness of fraud detection in finance. By utilizing advanced machine learning algorithms, DLSS can help financial institutions identify and prevent fraud more effectively, minimizing losses and protecting their operations.
The role of DLSS in risk management
- Enhanced predictive modeling
- The ability of DLSS to handle large and complex datasets makes it particularly useful for predictive modeling in finance. By using deep learning algorithms, DLSS can analyze vast amounts of data to identify patterns and trends that may be indicative of potential risks.
- For example, DLSS can be used to analyze historical financial data to identify patterns in stock prices, credit defaults, and other factors that may impact investment decisions.
- Additionally, DLSS can be used to analyze news articles, social media posts, and other unstructured data sources to gain insights into market sentiment and other factors that may impact financial performance.
- Improved risk assessment
- DLSS can also be used to improve risk assessment in finance. By using deep learning algorithms to analyze large amounts of data, DLSS can provide more accurate and reliable risk assessments than traditional methods.
- For example, DLSS can be used to analyze credit risk by analyzing data on borrower behavior, financial history, and other factors that may impact creditworthiness.
- Additionally, DLSS can be used to analyze market risk by analyzing data on economic indicators, geopolitical events, and other factors that may impact market performance.
- Finally, DLSS can help to increase efficiency in risk management by automating many of the tasks involved in risk assessment and analysis. By using deep learning algorithms to analyze data, DLSS can provide faster and more accurate risk assessments than traditional methods.
- For example, DLSS can be used to automate the process of identifying potential risks in financial transactions, allowing risk managers to focus on other tasks and improving overall efficiency.
- Additionally, DLSS can be used to automate the process of monitoring risk levels in real-time, allowing risk managers to quickly respond to changing market conditions and minimize potential losses.
DLSS in Education
The impact of DLSS on educational technology
Enhanced learning experiences
DLSS has revolutionized the way students learn by providing a more immersive and interactive experience. With the help of DLSS, educators can create engaging multimedia presentations, virtual simulations, and interactive visualizations that bring complex concepts to life. This enables students to grasp difficult concepts more easily and retain information better.
Improved student outcomes
The use of DLSS in education has been shown to improve student outcomes. With the help of DLSS, educators can personalize learning experiences to meet the needs of individual students. This helps to ensure that every student has access to the resources they need to succeed, regardless of their learning style or ability level. As a result, students are more likely to achieve their academic goals and reach their full potential.
Increased accessibility
DLSS has also made education more accessible to students with disabilities. With the help of DLSS, educators can create accessible learning materials that are compatible with assistive technologies such as screen readers and text-to-speech software. This enables students with visual, auditory, or cognitive impairments to access the same educational resources as their peers, and participate fully in the classroom. As a result, DLSS has helped to level the playing field for students with disabilities and promote inclusivity in education.
The potential of DLSS in personalized learning
- Tailored instruction: DLSS allows for the creation of individualized learning paths for each student, taking into account their unique needs, abilities, and interests. This approach ensures that each student receives instruction that is specifically designed to meet their unique learning needs, resulting in a more effective and efficient learning experience.
- Improved student engagement: Personalized learning with DLSS can increase student engagement by providing students with a sense of ownership over their learning. When students are given the opportunity to actively participate in the learning process and have a say in the content they learn, they are more likely to be motivated and engaged in their studies.
- Enhanced learning outcomes: Research has shown that personalized learning can lead to improved academic outcomes, including higher test scores and better grades. By providing students with tailored instruction that is aligned with their individual needs and interests, DLSS can help to enhance learning outcomes and improve overall academic performance.
DLSS in education has the potential to revolutionize the way students learn by providing them with personalized instruction that is tailored to their unique needs and interests. By incorporating DLSS into education, students can benefit from improved engagement, enhanced learning outcomes, and a more effective and efficient learning experience.
FAQs
1. What is DLSS?
DLSS stands for Deep Learning Super Sampling. It is a technology used in computer graphics that allows for the upscaling of lower resolution images to higher resolutions, while maintaining image quality. DLSS uses deep learning algorithms to analyze and improve the visual quality of images.
2. How does DLSS work?
DLSS works by using a neural network to analyze the input image and determine the best way to upscale it. The neural network is trained on a large dataset of images, allowing it to learn the characteristics of high-quality images. During the upscaling process, the neural network generates a new image that is a higher resolution version of the input image, while also improving the visual quality of the image.
3. What are the benefits of using DLSS?
The main benefit of using DLSS is that it allows for higher resolution images to be rendered without a significant increase in processing power. This is particularly useful in gaming and other applications where high-quality graphics are important, but the hardware limitations are a constraint. Additionally, DLSS can improve the visual quality of images, making them look sharper and more detailed.
4. What applications can DLSS be used for?
DLSS can be used in a variety of applications, including gaming, video editing, and virtual reality. In gaming, DLSS can be used to render games at higher resolutions, improving the visual quality of the game. In video editing, DLSS can be used to upscale video footage to higher resolutions, allowing for greater detail and clarity in the final product. In virtual reality, DLSS can be used to render virtual environments at higher resolutions, improving the immersive experience for users.
5. Is DLSS difficult to implement?
The implementation of DLSS can vary depending on the specific application and hardware being used. In general, however, DLSS is designed to be user-friendly and easy to implement. Many modern graphics cards and software applications include built-in support for DLSS, making it simple for users to take advantage of this technology.
6. Can DLSS be used with any hardware?
DLSS is designed to work with a wide range of hardware, including graphics cards and CPUs. However, the specific hardware requirements for using DLSS will depend on the specific application and the level of detail and resolution required. In general, DLSS is most effective when used with high-end graphics cards and CPUs, as these are better equipped to handle the demands of rendering high-quality images.
7. Is DLSS the same as AI upscaling?
While DLSS and AI upscaling are related, they are not the same thing. AI upscaling is a broader term that refers to any method of using artificial intelligence to improve the quality of an image. DLSS is a specific type of AI upscaling that uses deep learning algorithms to analyze and improve the visual quality of images.
8. Can DLSS be used for real-time rendering?
Yes, DLSS can be used for real-time rendering. In fact, it is often used in gaming and other applications where real-time rendering is necessary. By using DLSS, it is possible to render high-quality images in real-time, even on hardware that may not be powerful enough to handle the demands of traditional rendering methods.